Episode 55 Large Language Models: Myths, Facts and the Future of AI
Download MP3Audio file
LLMs_ Myths_ Facts and Future.mp3
Transcript
Hessie Jones 00:00:02
Hey there, this is Hessie Jones. And today we're talking about large language models. OEM's, as they're called, have emerged as one of the most powerful and most versatile artificial intelligence technologies of our time. So by training these massive neural networks.
On these vast data sets that that are human generated text, LMS are now ones that have developed this unprecedented ability to actually generate human like language and with robust fluency. And I would say comprehension. So this breakthrough has really unlocked a wide range of innovation and applications across many industries. But right now we're seeing things like content creation, language, translation and data analytics, conversational AI assistance, and even code generation. More recently, open AI released ChatGPT 4 O they say that it can reason across different modalities in real time, so they train this single model end to end across text vision and audio, meaning that all the inputs and outputs are processed by the same neural network. So it's still early day.
But this idea of developing a multimodal model has this potential to actually create more effective outputs and you will better decision making in the future.
So welcome to Tech Uncensored that the nacency of the technology has yet to be fully really understood – text, language, and audio understanding the generation capabilities that can drive substantial productivity gains and enable new forms of human and machine collaboration, and even question whether human jobs are replaceable–This is all still emerging so as well LM technology.
Currently have limitations. They have risks they have Issues when it comes to factual inaccuracies, they have biases that we've seen in the old guard of AI, from training data, they lack common sense reasoning, and this is pervasive potential for misuse and more recently the data privacy implications. As we've seen from open AI's unconsented use of Scarlett Johansson's voice.
So there are techniques out there like retrieval augmented generation, which some of the lot of the researchers call rag and they are highlighted as promising.
Coaches to help ground the LM knowledge and improve their accuracies over time, so I am so pleased to welcome Amir Feizpour, CEO and founder of AI Science, which is a platform to for expert in the loop business workflow automation, and I've known emir for many.
And he is just the right person to actually take us through everything about LLM's, and we'll cover the transformative impacts of this technology across the different sectors, the applications of both present and future. What are the risks and challenges to LMS?
And what does this mean for startups actually developing in this space? So welcome Amir.
Amir Feizpour
Thanks for having me, Hessie. I'm excited about our conversation.
Hessie Jones
So let's start with you, because you've been doing artificial intelligence for a while. So why don't you take us through your background you've also developed this amazing community of researchers and developers all over the world that have done some amazing collaborations in this space. Tell us how tell us how this all started and why you are here.
Amir Feizpour
Sounds good. So just to give you that, I guess your audience a quick history, I am a quantum physicist, planning I I did my whole academic journey of PhD in postdoc and all those good things. But at some point I decided to move to industry and the very first thing I tried was a quantum tech company. And you know, about a decade ago that was just too early.
And I decided that the next best thing was AI, and very much immediately fell in love with natural language processing because the ability to do computation on text was just crazy to me at the time, and you know when I saw it, I was like, what is this? How can I make my life about it? And you know, that's what I've been trying to do since I worked as a data scientist and then later a product person at one of the financial institute.
It was a fun gig, but you know, it was pretty bored at my corporate job. I think the part of being an entrepreneur was in me and one thing that I started doing on the side to entertain myself, but also to keep up with the rate at which information was being released in the space of AI was to build this community like and it literally started by a bunch of us gathering every week, eating pizza and talking about AI.
You know, that was six years ago and that's still going on now. We gather online obviously in a post pandemic world, but the same type of vibe still exists. We hang out every week talking about advances in AI build together, learn from each other and teach each other.
Hessie Jones
How big is your community at this point?
Amir Feizpour
So we have more than 5000 AI researchers and engineers globally, depending on how you count, but that's, you know, one of the metrics that we have.
Hessie Jones
Thank you. So let's dive into LM's right now we have the current limitations of, I guess, machine learning and whether or not I guess that's now considered passe. Does anybody say machine learning anymore?
Amir Feizpour
I did.
Hessie Jones
So then then it's true, then it's still here. So is it safe to say that LLM's are a natural evolution within the artificial intelligence space? And so tell me how they work.
Amir Feizpour
Yeah, definitely. So I mean when it comes to artificial intelligence, you know, one of the one of the very critical signs of intelligence is language use, right? Like if the if you look at the animal Kingdom, you know, we are one of the animals that has a very, very complex capability of using language and there's some aspects of language like a storytelling is specifically tied to cognitive revolution that happened to, you know, homophobia.
And so you know, as modern humans, we've been very, very interested in replicating that capability machines. Right. So like from fifty 60s, when we were interested in expert systems, we always wanted to say how can it talk back to me, how can it understand what I'm saying? Right. And, you know, obviously the.
Progress has been very, very slow but finally you know language models are like the large language models, like language models have been around for more than people probably realize. But the large ones have been around for the past three to four years, and they just completely, you know, brought the game to a new level.
Which wasn't a step function, changed like it was a gradual change over the past, you know, 3:00 to 5:00.
There's but, but yeah, definitely they have brought the game of modeling language to A to a very different level, although you know to to address what you said earlier, they don't necessarily replace a lot of the other types of machine learning that we've been doing like they're not necessarily good at time series forecasting and recommender systems and reinforcement learning. All of those other types of machine learning that we have been doing. So, they are like their own category that potentially interfaces with all other types of intelligence categories.
Hessie Jones
OK, So what? What specifically differs about them in terms of the technologies that they use?
Amir Feizpour
So there there are two important differences with with other types of machine learning technologies that we have developed previously. One is just the sheer amount of data they're trained on. Like I mean a lot of.
You know, previous types of machine learning that we have done. We usually had like a limited amount of data like it was the the largest data sets were the transaction data within this enterprise.
Or variations of that idea, right? Or or the watch time of YouTube videos. It was usually like super specific and narrow in terms of what the data was and what we could do.
With it but with language because of the variants that it has because of the abundance that it has, especially with the Internet, we have just so much data to train on, so that's one aspect that is very different. So the amount of available data that will still needed to clean it and turn it into high quality training data. But just the amount of available data was so large, which in turn allowed us to train very, very, very large models and these are pure enormous models and only very few entities can train, at least with the current architectures that we have, so that that's one aspect that we just see the size, the size of the data, the size of the model itself.
The second aspect of it is you know what it is trying to do. So the previous types of machine learning that we were interested in, they were generally speaking, single track ponies if you wish. Right. They're they're what we call narrow AI. So they're capable of giving you a bunch of data points about market predict where the market.
Going by the time series forecasting for the market or I show you what kind of videos Amir has watched, recommend what he would be interested.
Watch next, right? But with language models we are doing something slightly different, which is we're saying, Here is all the text from the Internet and all the code and all the data sets that exist on the Internet. Learn what's the likelihood of if I give you these few words, what's the likelihood of the next word and just learn this about the whole Internet. Right. And that's interesting because all that we are teaching it is if you see text.
This is the most likely next piece of text. If you see data, this is the most likely next piece of data. If you see code, this is the most likely that. That's sort of idea. That's what we call autoregressive training and and once it learned that it was large enough that all of a sudden it just code on code internalize a lot of patterns that existed in this. So that's why it can just connect the dots and be like I wouldn't write an article that sounds like Hessie Jones wrote it. I want to write an article that is like. I don't know Harry Potter, but Barack Obama wrote it right? So you can just extrapolate from all of these different places into doing it, doing the tasks that we're having to do. There are a bunch of other nuances like instruction, find that we can get into, but that that's really those, those two are the biggest differences versus the more traditional machine learning.
Hessie Jones
I think the one thing I want you to address is this idea that generative adversarial networks with that, because that that is that considered a a big component of this as well. In being able to generate this coherent text or outputs is Gans part of that solution?
Amir Feizpour
So gens were sort of the baby steps towards generative models, like the even the image based games mostly were used in image space just to generate images and even the early versions of them. They were pretty decent. But you know, they have a lot of problems and the current models do not use that Gan architecture anymore like there are diffusion based in text we you know, the conceptual idea, which is I'm going to give you a bunch of things and generate some result. And I will tell you what is good and what is bad. And the same idea does exist here. And you know the whole red teaming thing for example.
Is an example of sort of that idea taken and, you know, changed a little to say that you're a large language model. You're is responsible to generate. I'm going to try my best to break you, try your best to, you know, undo that, right. So that that's sort of where these sort of conceptual ideas overlap.
Hessie Jones
And so just for our audience, the idea of red teaming it is sort of a way to to create more accountabilities in the systems by by by trying to, I guess from from the outset, almost like fool, fool, fool. The network. Is that right?
Amir Feizpour
Yeah, essentially, you know you want your large language model to behave in a certain way. The idea of red teaming is like, you know, the training. Like you're a boxer. That's the training you do. Like you. You throw the boxer into a ring with somebody else. And you say just fight. You know, the goal is to, you know, make sure that your, you know, Boxer gets really good at predicting what kind.
You know, punches are going to come towards them and you know be good at reacting to those. That's exactly the same thing you're doing with Dell and you're going to say this is going to be in the wild. People are going to interact with it. You have no idea what they're going to do. So let's try all these ideas. Ask it to do all sorts of crazy things and make sure that the system is robust and can handle those.
Hessie Jones
OK, so let's let's talk with some of the big players here. We have anthropic. We have Gemini from Google, we have Lama from Meta.
And we have open AI like why do you think at this stage there has been so much interest in even adoption for these large language models?
Amir Feizpour
Use cases remarkable, right? Like the the number the number of use cases that rely on on a structured text data is huge, and that's generally untapped because of the narrow AI capabilities that we previously. We had we made a lot of progress on, you know what we call structured data like time series forecasting, recommender systems, anything you could put in tables. But on a structured data which is video, audio, image and text are things that you know started happening later, you know more recently. But language was the very hard one because we could get language to be kind. Of sort of. Close, but not exactly as good as these large language model we're able to do that, and the adoption is simply because everybody was so thirsty for having these types of capability.
Is and and you know if you think about them the way those types of capabilities are happening today is that we don't have any good way to automate them because they're very fuzzy. It usually requires A level of knowledge to know how to handle them. At least, you know, language. Knowledge to read something and react to it, and all of a sudden others are giving us those capabilities. So you know, we're we're able to consider for the first time. Automating those things I've been trying to throw around this term that I'm trying to spin called knowledge OPS, which I think is a continuation of, you know, Dev OPS, data OPS and Nops. And it is knowledge OPS. It is really, you know, operationalizing knowledge intensive tasks that were not available before this.
Hessie jones
OK, so I want to get into the the point about these large language models like how how they're generated and we know that there is indiscriminate scraping across the web. I don't think. I don't think anybody ever thought about the privacy implications of just taking the web and saying I'm going to put it all into all these Data sets that we're going to train from so address the idea of There's only a few companies that have the ability to scrape the Internet and develop models from that. From a cost perspective. So what happens with other companies who use, let's say the API's from these models and they fine tune them for themselves?
What is, I guess, even from an ownership or control perspective, how does that work in?
Amir Feizpour
The new world. That's a very unresolved issue. Like we are in very, very early days of figuring out how this might work. You know, probably a lawyer is or a copyright person is is in a better place to comment on what it should look like. But currently what is happening is.
Essentially, you know, as you said, they've used data from the Internet without necessarily even asking. Can we, you know, the very good old trick of, you know, ask for forgiveness and. And, you know, they are now trying to ask for forgiveness in a bunch of lawsuits that he's, you know, gone through, that they're going through.
But you know the the fact is that they created like open AI specifically created a model that that was the first product product market that you know that the 1st 2 product markets that which means that you know that they have their attraction.
Which in turn means that you know they have the capability of generating a lot of new data that is not necessarily even available on the.
Like famously their, you know, human feedback data was acquired like they just hired a bunch of people to annotate that data, right? Even, you know, underpaid from.
I heard like I I don't know if that's true or not, but I I do. I have again heard that they're actively hiring data annotators like people, that you would sit down and solve puzzles and write your reasoning or any type of, you know, human activity. You know, I wouldn't be surprised if they're hiring people actively to sit down and annotate.
Therefore, those kind of things, right?
So they definitely have the momentum, resources and technology to collect data at the rate that nobody else can, so that that's definitely concerning. But at the same time, open source has not been, you know, inactive either. Like you know, we're seeing a lot of open source models.
Some companies, for whatever reason, like, you know meta for example, are open source and a lot of the models they're building.
Which is an interesting play. And you know again like I don't have the knowledge to know what is the politics of doing so, but at least the fact is that it is enabling a lot of the community to keep up and build independent models and fine-tuned independent models. So the question of you know, what's the structure of the ownership is going to look like you know what's the right economy around it? Well, that are very interesting questions and I don't know if you know answers to them. But what I'm hopeful about is the fact that like one potential outcome could have been that everybody kept the models close to their chest and said nothing in the open source and you know, no open source project was supported and that would have been really bad. But at least the fact that there is activity in the open source community and at least some of the Community companies feel that it is important to support those movement. I think that's positive. You know, hopefully there will be some forces in the market that create a New Balance that is better for everybody.
Hessie Jones
There's actually, I think, a friend of mine who works with, I believe, the Biden and Harris government, where they're trying to do this. I think it's the, it's the executive order for for AI ethics. I don't know, it's called AI ethics, but the idea that they could potentially audit you as a company so that you have to be a little bit more open about your model, that it regardless of which model you end up.
Thing, but that it it's supposed to do what it was intended to do, and it's also open to testing to ensure that there aren't any potential downstream effects. I think that's coming.
Question is whether or not those companies that have been black boxes will will be forced unless legally they're forced to open up the hood and to be more transparent about about these things.
Amir Feizpour
Yeah, I mean I have a bit of a cynical point of view about regulation in general. I I don't find it very effective.
The challenge with regulation is that it's usually several years behind where it needs to be and very, very slow to be implemented and more importantly, companies know in and out of how to get out of them right and just make their appearance look right and move on. So think what what the saving you know approach might be is just to enable entrepreneurs, you know at altitude accelerator. That's exactly what you're doing. But just entrepreneur entrepreneurs or ambitions enable them to build alternatives. And make those alternatives available on terms that are more suitable, right? Like create a competition at a company Vision. He's going to create a New Balance.
Hessie Jones
And moral, right? Yeah, I think I think so. But there has to be. You have to instigate a little bit morality or, you know, just because I I mean, at the end of the day it's that it is, it is innovation. But we structure the innovation so it could be more accountable.
Amir Feizpour
Absolutely.
Hessie Jones:
So let's get into some misconceptions about generativity I'm going to throw a couple things at you and you tell me whether or not it's a myth or fact, and why so LM's can think or reason independently like humans.
Amir Feizpour
Yeah, that's one of my pet peeves. I've been talking to a lot of, you know, very knowledgeable like, you know, mathematics PHD's and such about this topic. I don't think there are good answers. My position is that they do not because, you know, they're based on statistics and statistics, by definition, is not causal and in order to be able to reason you need causal reasoning, right? I think they're very good reasoning. Parrots like they've seen enough text on the Internet that looks like somebody's reasoning through a problem, and they can pair it. That, but the fact that they hallucinate is a very, very good indicator of saying ohh if they have not seen enough reasoning of this type, they're just going to hallucinate that reason. So yeah, that's definitely not the.
Hessie Jones
Got it.
Case that's good to know, though, that's that's actually good to know that. They're not ready.
I would hate for for the 1st Integration of ChatGPT to say that they know exactly what Hessie’s going to say next. Right. So OK, so our LM's unbiased and objective.
Amir Feizpour
They are as unbiased and as objective as their designers, right? So like very much like all other machine learning models, they're going to inherit.
Biases from their data and from their design. So for example, one of the things that gave open AI an edge very quickly was was the, you know, quote UN quote alignment with human preferences, but which humans, like humans that open AI said you're the right group of humans to, you know, provide preferences for the rest of the humans.
Hessie
OK, so LLMs can generate human like text. They are truly creative and innovative.
Amir Feizpour
I think the first part of that is correct in a sense that they are probably better than 90% of people in writing. You know, grammatically correct and coherent text.
The true creativity. I have a hard time with. Uh, you know, even like things like, uh, mid journey and stable diffusion and such. You know, the majority of the creativity comes in pages and pages of prompts that somebody writes like that, that that the content of that prompt is creative. The rest is just the model, you know, replicating what it is told to do, and I think that's the same with language model.
Hessie jones
Yeah, I I found that like I had to on mid mid journey that generate the logo that I wanted. It ended up taking about 10 to 15 prompts to get it to where I wanted. It would probably it was cheaper because a full year license, but it for from the perspective of time.
I probably would have gone to somebody on fiber who probably could have developed it and half the time because they I was prompting what I wanted when I I completely did not rely on somebody who could have done it outside of the box.
Without my interference. But I think that there's some trade offs
OK, LLM'S can continuously learn and expand their knowledge like humans, and I think you already. You already said this one, but go ahead.
Amir Feizpour
Yes, if you have it lot of lot of money because you have to keep retraining them right, like and you have to keep retraining them from scratch.
Because they have this catastrophic forgetting problem, where if you just throw in a little more data and try to retrain it, it's just going to forget some of the previous information. So the the most robust way to do that is to retrain it from scratch and nobody has that amount of money to just keep retraining these. And there are a lot of other approaches. Retrieval or augmented generation as you mentioned. But yeah, the models themselves, it's very like that's that's why open AI is keeping the model from 2021 and not updated because it is.
Hessie Jones
So explain the how retrieval augmented generation and how it works.
Amir Feizpour
So that's essentially the the easiest way that I like to talk about it is separating the knowledge base and the linguistic interface. So essentially you have to admit right away that the language model is just the linguistic interface. It does not have knowledge. It should not rely on its knowledge and separately the data layer is where the knowledge exists so that data layer could be actual. You know I don't know structured data, it could be documents, could be anything like that. So the role of the language model becomes has he said this question that is what is what you will augment the generation. Therefore I'm going to make a query against these documents.
Figure out which paragraphs have a description that answers this question. Bring them back, put them in the context of the LLM and ask it to answer that question only using this text. So that way you're using a reliable set of documents as your knowledge base as your database, and the role of the LLM is pretty much understand what Jesse said and then provide a good answer based on these pieces of text that I'm giving you.
Hessie
So, basically, now getting to more fine tune the model specifically for stuff that you know that's relevant or I would say like a good reference point for for the.
Amir Feizpour
Yeah, so fine tuning as a term is something else, but you are essentially grounding it into a knowledge base and that's very important because there is also a lot of nuances around like when you're separating the data and the linguistic interface, very much like a normal website where the data layer is separate than the UI.
And then the reason is that then you can put things like privacy control, access control, you know, removal of PII, and between these two, that's exactly what you can do here. Now, right? Because you can ask me a question that you're not allowed to know the answer to. And then this intermediate layer can just reject that question and say, hey, you're.
Not allowed to ask this question.
Hessie Jones
OK, got it. OK.
So the last myth or fact LM's are deterministic
Amir Feizpour
I think that everyone has seen already that you asked the question twice and gives you 2 answers.
It's probably, I think, one of the reasons why if you end up building anything, even from a a stronger compliance based, let's say in healthcare, that if it is deterministic, that's a good thing in a way because now you can you have a better reliance on what the output.
Could be, but because of the way it is right now, generated AI is not even being considered as as part of, let's say, FDA approval or anything like that, right?
Yeah, I mean there, there, there are few. There are a few ways that you could get it closer like there this parameter called temperature which you can reduce which makes it more predictable not deterministic but more predictable like grounding it into external knowledge source like right. Does that makes it more predictable.
You could create verification modules that check the output and ask it to try again. If it does something incorrectly.
Though or, you know, they're also, if you're creating something custom, you could also instead of asking it to generate one response, you could ask it to generate the view and then do a majority vote there. There are ways to make it more predictable, but definitely deterministic is nowhere close to where it is.
Hessie Jones
Let's talk about some of the issues when it comes to generative AI, I think one of the biggest things that I I'm finding and especially between the if you try to separate the haves from the have nots based on the size of the tech company, there is a capacity and not knowledge limitation. Can you talk a little bit about that?
Amir Feizpour
Yeah, for sure. I mean the, the, the game of very, very large models is just one by the big entities because that's where the resources compute the resources are to run these models.
But that's not all. Lost for smaller entities like startups, because one one of the misconceptions is that all of them can do everything. You just need to throw the right data at them like that. That's definitely incorrect. So you still have to do a lot with the right, you know product development, steps of figuring out the right problem, figuring out the process for a design for that process, create the proper guard rails around that process for the software to work in, integrate it into the work so it leaves a lot of room for startups to go out and figure out.
All of these details, which means that sometimes you might not even need the largest model, sometimes for your use case, maybe a medium sized model interface with a bunch of smaller models is a much better plan.
So the I think the knowledge gap, yes there is a gap between available resources, but the opportunity I think is is elsewhere the opportunity is in the good old business bottom line which is if you're creating a startup go out, find somebody with a real problem and figure out how to solve the problem.
Hessie Jones
So you're basically saying that we don't have to throw out the old and assume that the new thing is going to replace it. OK.
Amir Feizpour
Absolutely.
At least not not in the near term.
Hessie jones
Now in your your term. OK, so let's talk about synthetic data. OK? And so there's an increasing reliance on it for, for training these large language models. And so I read something about potential model collapse and the degradation of the quality because of because now we're.
Creating new synthetic you we're we're replacing data with synthetic data. Can you tell us more about what those impacts are?
Amir Feizpour
Yeah, for sure. I mean against the terms of service of most of these large models, a lot of the smaller open source models are trained on the output of the larger models.
Generally speaking, there's this concept called knowledge distillation where you could you could grab a very large model that does some thing and then sort of distill it to a smaller model that you know repeats the majority of the behavior of the large model but is more specialized in how it does so. So because of this dependency, there is a bit of a concern that you know, depending on how the large model is behaving, the behavior of the smaller.
Autos might be dictated and constrain. So and you know, if you just keep doing this for for a very long time, eventually it's just a closed loop like there is no not enough diversity. Which might or might not be true like I think the the opportunities that exist in the near term is is going to be all around.
Essentially, all the data that is not on the Internet, so like from a research point of view, yes, you know that there is a ceiling potentially.
But from like a business opportunity point of view, there's so much out there and most importantly becoming very creative in creating an MVP that allows you to create your own data sets. Is the name of the game that's the most important mode you could have because.
You know the rest of this stuff is what everyone has access to, all the things on the Internet. Everyone has access to even the data that that is owned by businesses. You could imagine that a competitor startup is going to, you know, come up with a deal with them and get access to.
Same data. What will be super unique and most like for startups is always going to be the data that you can collect yourself. And you know, in principle that's limited by your imagination, not really anything else.
Hessie Jones
OK, so let's talk about. Let me see what one of the other issues that people are worried about is OK, amplification of biases. As long as humans are involved, I would assume that biases are, you know, commonplace.
Amir Feizpour
Yes, but you know having machines do it is also not necessarily going to change that because you know they they're going to repeat the biases in data and the design.
So the the only answer is, you know, people sitting down and thinking carefully about and. But this is not always a bad thing like training, training is creating.
This so like, you know, having an inventory of all the all the biases and then you know, a taxonomy of these biases are bad. And we don't want them. These biases are good, but this 99% of biases are sort of Gray. So what do you want to do with them? Right, like the gender gendered language.
For example, a very good example that I like to talk about in this context, because English does have German language but a lot of other languages like Turkish, Farsi, etcetera don't. And when you translate from one to the other it says you know she's in there, she's a doctor. That's the canonical example. But you know, maybe that's right. I mean, in a very specific situation. And she is the nurse. He's the doctor. So, like, what is the right product design? What is the right policy on top of this translation that allows that adaptability that says OK.
Well, are we talking about a woman or a man in a translation? Right where the system should be. So essentially what we do with biases is a very non trivial thing for a lot of kids. Like there are some biases that are very clear are Bad. Some biases are very clearly good. Like, you know, alignment with general human preference. That's a bias we're creating, but that's a good. What to do with the other? The Gray area ones? Those Are the interesting questions.
Hessie Jones
Could be cultural. That could be that. Yeah. Their whole host of other values that you need to take into consideration. OK. So for let let's turn a little bit to startups who are considering using these LM's for their own purpose.
Amir Feizpour
Absolutely.
Hessie Jones
From your perspective, because you work with a lot of startups who are starting to use LLM.
What do you think are the things that they need to consider in determining whether or not that, first of all, are they going to use this, or are they going to use, let's say, like you said, some of the traditional AI machine learning and what do they have to think about when, when, when they decide to make the switch?
Amir Feizpour
Yeah. So I mean not skipping all the good practices is is the baseline, right? Like you cannot escape.
Getting out there and talking to customers. You cannot escape the the right, you know, product development steps. You cannot skip the right software engineering steps like you have to do all the above. And that's like 95% of the game. Still the thing that is different is that now you have one new level block to put inside.
The system you're creating to solve with specific customer pros And that new Lego block has a bunch of new capabilities.
So in all likelihood, though, whatever you end up building for a specific customer problem is not going to be just one model. Like, you know, all likelihood, it will be probably 1 larger model, a bunch of small models, all of them interacting with each other, and the IP will be really about how these are.
Architected to work together to interact with each other, to control each other, to scaffold the behavior of the large language. And to verify that God reward.
And then you know what data is supporting both The system right?
And because you know the data that is available on the Internet is available to everybody. So what is your unique mode data mode? So again, like none of these are really new like all of these have been around forever. It's just been systematic, principled.
Evaluation driven and really knowing what is it that you're trying to improve.
Are they? Is it going to be different executional when you are start to switch to large language models because a lot of it is prompt based as opposed to natural programming?
Ah yes, I know. So a lot of better, a lot of the better models are a lot less sensitive to the exact details Of the prompts.
And a lot of well designed systems remove the burden of prompting from the user and bake it into the back end to write the prompt. So a lot of the systems we design, we spend a lot of time understanding if the user says this, they might mean these things and they might need these types of information.
So the prompt is written automatically in the back end that goes to the like between by child DVD. Is it is a bit of a weird product because the distance between what you're writing and the model is very short.
In most proper, robust software products, that distance is much larger, like what you write is analyzed and added and expanded, and like write is a very good example, right? Like what you write is not the prompt, so it does still take a lot of software engineering to create a modular system that automatically.
It's the right prompt. But and that's like 95% of the game, so about 5% of it is still, yes, like the back end has to have the right system prompts and the user has to. And that problem will reduce overtime as we include more data into the prompt about the user. But in principle, yes, it will still depend to some extent on you know how the user is saying things on the interface.
Hessie jones
OK, it seems to me that maybe this is a separate discussion about whether or not there is vulnerability for the software developer over time.
Vulnerability in terms of job loss.
Amir Feizpour
And there is vulnerability everywhere. What I have is a hard time wrapping my head around though is the whole conversation around job loss, I think is a little misguided and emotional. I think what is more likely to happen is the job description loss, you know, like so far you only needed to write in Python. Now you need to write in Python but also be good at you know English.
Or maybe you're a you're a business person. So so far all you did was write in things in English. Now you need to write it in in a very specific way that the model understands right? So these types of you know the the details of the jobs are going to be fluid and change but, you know, just wiping the whole job overnight, I think likely to happen. So the big question for me there is how can tools like these that are automating some tasks within these jobs be used to then quickly upscale me to do something different that I wanted to do but I was not able to do before.
Hessie Jones
OK, got it. So that brings us to your last question and it's a good segue from your last comment because this is we're talking about a technology that's going to be embedded everywhere in business, in our daily lives and how we communicate with each other.
So we're philosphically and you and I had talked about this just beforehand. What is your sense about dependence on the technology that's going to emerge in a way that has, I don't know, where it has implications on whether or not we can control it? That it can behave as as intended and whether or not you know humans will make the right decisions. But as it as it's as it's being built out into society.
Amir Feizpour
I mean it is. It's a, It's a very valid and deep question, and it does have societal impact, right? Like I think as one of the topics that you know, I I talk a lot about is you know, the agentic workflows and multi agent systems.
And I think they will result in a lot of redefinition of work, right, because as as we just said, the job descriptions are going to change, they're going to turn into new job descriptions. But I don't think it's going to stop there. It's going to just change organizational structures like, you know, if a lot of the work that middle managers.
We're doing today or that cognitive handling of, you know, transferring the business metrics into specific tasks for, you know, the groundwork.
There's, you know, would we need them anymore? And if not, then what does it mean to. If agents become very good at hiring gig workers to do something, onboarding them, having them do it, and then off boarding them, then what does it mean to have them have the boundaries of of, you know, corporate? So there are a lot of very, very interesting.
Impacts on the future of work, I think specifically, but also in societal impact in general as you said.
I have a hard time, you know, obviously it is very difficult for any of us and I have a very hard time predicting where it will go. But you know, my bet is still going to be on founders who are enabled well by, you know, money, capital and you know, connections and influence to build guardrails to build, you know alignment modules to build privacy by design to build, you know, responsibility by design, and that's the only way that I think we have a chance of, you know, controlling where this will go or at least reducing the potential bad side of it because anything else that we would do like regulations like relying on corporations, none of these are going to be that reliable in terms of creating the right balance to end up with the right outcome.
Unless you create an LLM specifically for governments that will accelerate their operations in even their thinking, maybe just to give them, hey, OK, hit me up on what happened in the last 10 years.
So that that I can catch up to how to build an LLM?
Amir Feizpour
That's the the reality is, is that I wish.
Hessie Jones
If government had the funds to invest in in smart people, the way tech does, then maybe they would have the controls that they need in order to to really fully understand how to how to legislate this technology. But that's the unfortunate part. And I think there's that there is an imbalance.
There, it's capitalism that's that's taking over, right? So. Anyway, any, any last thoughts? This is amazing.
Amir Feizpour
I wish I could talk to you about These types of topics, you know, we can definitely talk about them for days and days and days, but you know a lot of the, you know, societal Structure that we have around governance, around work around, you know, social interactions. I think a lot of these are going to fall apart. You know, as these new technologies, you know, get more and more embedded and integrated into how we work and how we live.
You know, obviously as you've highlighted a few times, doing it responsibly is very important.
Not bottlenecking that on one entity is very important, especially if that entity is large and slow to move. Like you know corporations or you know government. So I I'm very interested and always excited to you know have discussions around.
What does this actually mean? Like what are practical, realistic ways?
Of you know, controlling where this is going to go, and I don't think control is, is is also the right, the right term to use like I think really what we're looking for what you're hoping for is you know reducing the harmful size and amplifying the useful. Wise right? Because the same technology. Yes, it can wipe out humans, but it could also potentially be an equal Using force in, you know allowing people to have access to, you know, building companies that they never previously had because it was way too expensive for them to hire engineers and product people and. And so I think that that's really the balance that we have to figure out how to get right, like how to empower the good use cases and dampen the bad use cases.
Hessie Jones
Thank you. That's amazing. Thank you for that. OK. So I think that's all we have time for today and I I thank you so much, Amir, for joining me to discuss this important topic. And I think for the most part, this is this is a topic that many people really need to understand because it is now moved beyond the AI community to now like basically society, so I will make this available to everybody who wants to really understand this technology itself.
And how it's going to impact their lives in the future. So thank you. And for everyone else, tech Uncensored is powered by altitude accelerator. It's produced by Blue Max and Transistor radio. My name is Hessie Jones and I am your host. And until next time, have fun.
And stay safe.
Amir Feizpour
Thank you.
Creators and Guests
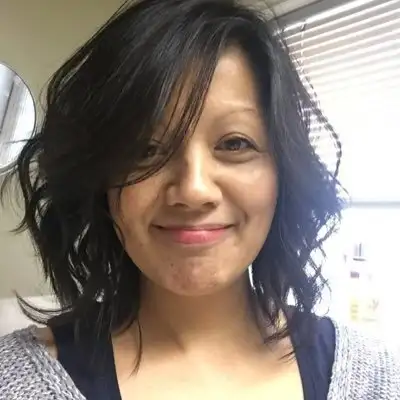
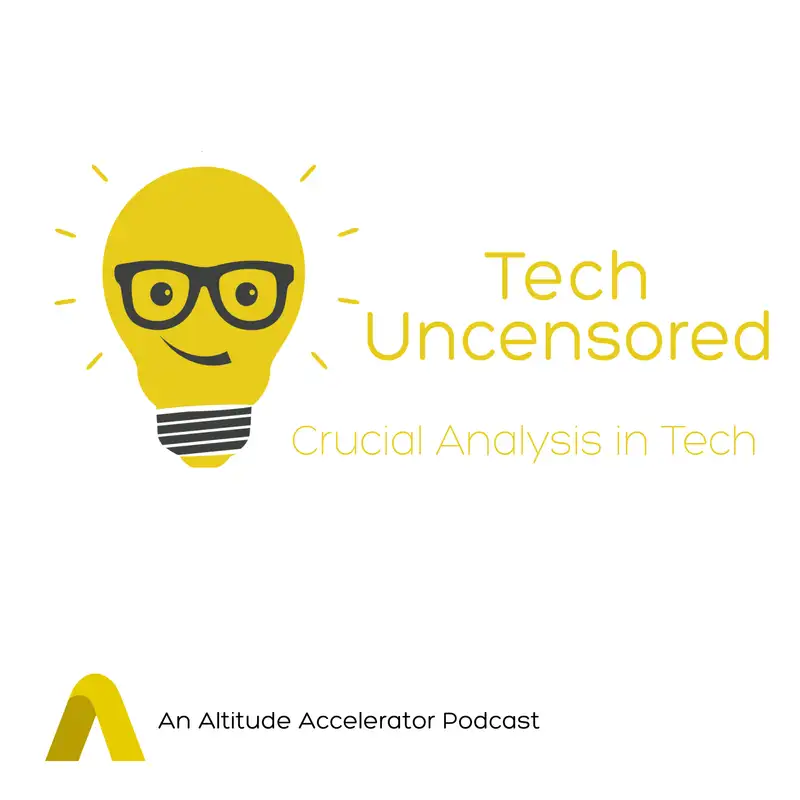