Episode 36 Has AI Robotics Revolution Met Its ChatGPT Moment? A Chat with Covariant CEO
Download MP3Hessie Jones
Hi everyone, this is Hessie Jones and welcome to Tech Uncensored. Today there are about 3,000,000 industrial robots around the globe, roughly 400,000 of them are new robots that enter the market every year. And the global market. Is expected to reach almost $43.8 billion in revenue this year. The growth in North America from a CAGAR perspective is expected to reach almost 10% through to 2028 so there are a lot of startups already developing robotic technology to help factories address some of the labor shortages and mitigate future supply change, chain disruptions. And so today, I'm really excited to speak to Peter Chen, who is the co-founder of Covariant Brain, one of the world's leading AI robotics companies that's actually modernizing today's warehousing, as well as changing the future of e-commerce. So Peter and his cofounders are at the forefront of AI advancements since they were researchers at Open AI. So, we're going to discuss a little bit about his journey pre covariant and it's actually funny, I actually said pre covariant. It sounded almost like COVID. So they welcome Peter and thank you for coming today.
Peter Chen
Thanks for having me.
Hessie Jones
OK, so let's let's talk about your your beginning. So you're one of the researchers at open AI. Before starting your company, tell us a little bit about your experience and actually advancing AI beyond what we consider today actually could have been yesterday machine learning and what was the vision for the company when you were there?
Peter Chen
Yeah. So I joined opening up pretty early like joined opening it when it was about 10 people and then left when it was about 100 ish. And the first time that I joined open AI Open, I didn't even have an office back then we were working out of Greg Brockman. The CTOS own house in San Francisco. Over there was definitely a very magical place from the very beginning. Like you, it has collected a group of extremely ambitious and talented AI scientists and researchers to think big. Like you have a lot of research labs around the world that work on interesting stuff. Open AI was one of the very few that really was thinking about what the big Leap is forward in terms of general intelligence. And it's really that mindset that has helped created this big movement behind team and this like. Really, the AI revolution that we're looking at today and we are covering building AI for robots, like really adopted a lot of the same philosophical, cultural as well as technical approaches that sit behind. Power today, which I can talk a little bit more about and. There's actually a shocking amount of similarities between trajectory in the language domain and covariant brain in the robotics domain.
Hessie Jones
OK, before we get to that, I'm interested to hear about whether or not you're surprised by the level, I guess of adoption in such a short amount of time and was this something that when you were building it year years ago I mean what was the expectation for what you were building and to what extent it would be ready for mainstream?
Peter Chen
I would say they're really two. It's actually not just one surprise like the four surprises how quickly technology has advanced. And then the second surprise is how quickly it became adopted, but they are somewhat related like so if you look at the core idea of chat reporting like or like, really, it's more fundamental piece, GPT. The idea behind it is relatively simple like 1 core idea. Is this idea of training a foundation model as opposed to a task specific model, right? So like what I mean by foundation model is that an AI model that is trained on many, many different tasks so that they can learn across all of these different domains and tasks such that it generalize to the next one better. And this approach sits at the core of ChatGPT, right. It's trained on the Internet of text plus textbooks plus Wikipedia, plus opening its own proprietary data sets. And it's by training on all these diverse set of data which really is representing different language tasks. Right. It's the ability to learn across all of these using a foundation model approach that makes it so powerful.
I would say like that this existed multiple years ago and I'm surprised that this direction works, but I'm surprised by how quickly it has advanced to the current day capability that it has. So that's the first surprise. Like, I'm not surprised that it would happen. I'm surprised by the pace of it. The second aspect is how quickly it became adopted. Like I'm definitely surprised by just how quickly. Almost everyone that you talk to in tech in general professional world has heard of or have used it. The core reason behind that is actually connected to the first technology point that I'm making. Like is this approach on training a foundation model so that like the AI works out-of-the-box for new questions that you want to ask it for new task that you wanted to solve that makes this. Rapid adoption possible, right? Because everyone, when they use creativity, they have different kinds of tasks that they want to continue to solve, like they have different problems in different domains that they want to attribute this of like part of the reason why ChatGPT has the ability to gain popularity and adoption so quickly, is because it works on a lot of the tasks that people ask it to do, even if those are things the AI was not specifically trained on right. So it's really this rapid advances in foundation model that gives us the ability to generalize to new tasks better. That gives us this foundation for people to adopt it quickly because it actually works for their tasks. And it's important this kind of capability becomes so normalized very quickly in the last couple of months. But let's take a step back and think in the general scheme of AI, this has been extremely unusual, right? Like before, this type of foundation model approach to AI. We have AI before, right? But they were just task specific AI, right? Like you have AI model that's doing useful things, but you need a group of AI scientists to come up with a task specific AI model and you need to collect data specifically for that task. And you need to go through expensive training to actually produce an AI that is good for that task. If this makes it very hard for AI to gain mainstream adoption, right, like let's say. I have a specific task that I want to solve like I personally definitely cannot afford paying a team of scientists to come up with a specific model and collecting a bunch of task specific data so is this huge shift from task specific AI to general AI that is powered by this foundation model approach that makes this rapid adoption possible, and this is in this idea, is where I would say there is a very interesting analogy between AI in language and AI in robotics, right? Like if you think about the previous shift moment, there were a lot of usage of language related AI already, right? Google Translate sentiment analysis? Spam detection, summarization. These were all language AIs. That has existed ppre–ChatGPT but what you have seen is that once you actually have a general AI for text that is ChatGPT, you can certainly open up so many more applications and so much more value that completely eclipse. The magnitude of AI usage in language before and this is going to be the same thing as what is going to happen in robotics, right? As you have cited, robotics is already a huge market that is growing pretty quickly, but it's actually still this miniscule version of what it will be like once we actually. Have that general AI for robotics likes so what we're really seeing now is like what I would consider the pre ChatGPT moment for language AI like we actually expect to see a lot more explosive growth like as we view this general AI for robotics in a way that is similar. To ChatGPT and that's what we're doing at Covariant Brain by building this covariant brain that is the foundation model for.
Hessie Jones
OK. So let me ask you. So what was the impetus or the influence that made you shift gears and leave open AI and start.
Peter Chen
There could be reasons like, I mean overnight at that time, still was a very research focus and not as much product focused company. When my cofounders and I left open AI and there was a great beauty in that approach, at that time we just believed a better way to build this general AI technology is by actually building products and deploying systems into customers, real production environments and collecting data, learning to feedback into the model building. So that's the approach that we had taken back then and. I mean you arguably, say, open AI has since then taken this more product approach as well, right? By launching the public API, then later transcript product, which is all a way to bring a productized version of the AI to the market and use data and feedback in real production usage to improved AI. But when we left open, it was not like that was not the direction of the company back then?
Hessie Jones
OK, so I I would say that robotics it is, it is still very nascent, although I do I have seen some amazing, I don't know if they're called prototypes coming out of Boston dynamics like some of that stuff is amazing and I think a lot of it's still used it's still being I guess implemented in some warehousing systems, but I want you to help us understand this notion of modern robotics and how it's different than it was, let's say, a decade or two ago.
Peter Chen
So I would say robotics has been always a backbone of modern manufacturing, but robotics in the past have been limited to only doing repetitive movement like you program the robot to do one thing and then it keeps doing that again and again like and so you need your environment to be perfectly structured and you cannot have any changes in what the robot is able to handle because it's only able to repeat the same motion. And what modern robots, and really what we consider AI robotics. Is really taken take that same really industrialized hardware that is really good at highly accurate motion but different brain. Give it an AI brain that actually have the robots. Understand what's happening around it and make decisions and act differently, like when your surrounding environment changes and this is going to unlock all those of magnitudes more use cases for robotics, right? Because if you think about what's happening in our world, there's only a tiny fraction of things that you can solve by perfectly. Repetitive motion like even when we pick up our phone like depends on like where you place your phone. You're going to exercise a different motion to do it, like you don't just do exactly the same thing again. And so, there's just orders of magnitudes more tasks that are currently untapped by traditional robotics, because robots only used to be able to repeat things. But you actually want robots to have a brain to see the world and understand it and make a different movement every time, and that's where you need really AI to unlock the next explosive growth of robots and the covariant vision is you have one foundation Model 1 General AI platform that can empower robots. Across the world, in different facilities during different tasks to handle the world intelligently.
Hessie Jones
There's a quote by one of your investors that I think is interesting because this is what you're alluding to. And he says “developing an AI system that can operate a robotic arm accurately, then identify things from a pile of jumbled, I guess, goods. Upside down, sideways leaning, and then get it right. He goes. That's extremely hard. So from what you're saying, I mean, if we were in a perfect factory and things were going down a conveyor belt and everything is measured to an, I'm just thinking, like peanut butter or cookies. That's the system that we have today, that that's fairly accurate, but what you're talking about is that that system cannot subsist long-term. We're still going to have to deal with anomalies and maybe these anomalies become kind of like the mainstay, because there's always human intervention within a factory setting. Is that right?
Peter Chen
So when you're when you're getting at this kind of like the core of what makes this a challenging AI problem is, is the change is the normally is the diversity of scenarios. They need to handle right like and this is like a big reason why we start. But developing covariant brain within warehouses and the logistics space is there's constant change like your you have new products being introduced to a warehouse every day. There's new packaging, there's even the same packaging that can be damaged, and you can have multiple different kinds of items together and will always change new things that a robot needs to understand and solve in front of it. And this is where the challenge comes in. Like you have huge diversity of scenarios that need to handle, and you need to be able to handle them with a really high degree of autonomy, right? Like you need to handle them with high throughput. You need to be able to handle them with really high accuracy and those are tough AI challenges and covering very and being a foundation model allows us to learn across all of those hard cases across whole industry sectors, all the way from pharmaceuticals to cosmetics to fashion apparel to grocery, like the ability to learn across all of these different places, gives our AI a general understanding of the world like so the next time that he sees a new item, a new circumstance. Even though this exact circumstance has not been seen before, like it's seen a lot of things that are similar to it before that gives it the ability to handle that new situation out-of-the-box.
Hessie Jones
OK. So tell us about it. I guess the next question is probably a question you're probably you've expected because everybody worries about the next generation of computers and or robots that are going to replace real human work. And do you have a different point of view in terms of whether or not that's actually a casualty to the human race or is it an opportunity?
Peter Chen
We at Covarant Brain are strong believers that robotics is a huge positive force to us as society and us as human race. The reason for that is you don't need to look very far, right? And you look at why do customers adopt AI robotics like they adopt AI robotics because they struggle to find people to do the kind of repetitive and injury prone tasks that exist in these warehouse environments, so if you look at the actual Labor Statistics in these workplaces you will find that those jobs have oftentimes more than 100% annual turnover rate like so meaning on average like every single work location like you will see a new person there, right? And it's that extreme turnover rate that signals how difficult it is to find people that want to do those jobs and how difficult it is to retain people to do those jobs. And so AI robotics, we have found to be a great way to help workers alleviate a lot of those extremely repetitive and entry point tests that they don't want to do and upgrade the jobs into something that's a lot more fun and engaging, which is supervising the fleet of robots. So as opposed to having to go there and do the same thing thousands of times a day like now, my job is actually to oversee a team of robots like and help them figure out the optimal way to use them. And so that's the type of thing that we can do and very narrowly like to the customers that we have and the people that work there and If you zoom out and think about the broader view of the risk that AI has imposed on human, I would say robotics is actually a very safe form factor to use AI compared to like an arbitrary AI that can browse the Internet, execute arbitrary code and ccan-doarbitrary things on the Internet, right? If you think about AI robotics, especially in the warehouse world, you have a very natural boundary of what that AI will do or will not be able to do. Like we're talking about industrial robot arms that's operating in a fixed footprint. With safety cages around it and so. There's a really safe by definition, use of the technology that is different from say an arbitrary language AI on the Internet that can do anything, and we also believe there is significantly lower risk of technology misuse, right? Like when you think about like all these type of generative AI technologies, a biggest risk of them is misinformation, right? Like if you can use these to generate misinformation on text on fake videos, fake audios, fake pictures, there's like real technology misuse risks and those don't quite translate to and again like this comes from because the intelligence, the AI is channeled through in a much more confined the boundary that is designed to produce productive work and designed to minimize any risk and damage?
Hessie Jones
OK, so where do you think we are today? Because you're talking about, let's say AI robotics being trained generally across many, many different scenarios. And ChatGPT obviously has a not so many use cases across billions of data points. That robotics is not necessarily in that same boat in terms of the aggregation of the number of use cases. So realistically like where are where is the industry. At this stage and to, I think, to quote you, when is it going to have its ChatGPT moment.
Peter Chen
It's starting now, right? Like when we look at where we are deploying robots into like we are seeing the same robot brain being successful with multiple cosmetics customers with multiple apparel customers with. Pharmaceutical customers with multiple grocery customers like these are all robots that are doing very different things across the world. Like like these robots are. Some are in North America, some are in Europe and they are networked together and then learn together, right? So like I would think.
Hessie Jones
Can you give an example of let's? Say beauty industry. Or retail. How? How or what kind of tasks are these robots doing?
Peter Chen
Yeah. So these robots are handling various kinds of manipulation tasks, like tasks that require your hand to handle. Like so that could be. Picking up something, inspecting it, picking up something, and then sorting it into different customer orders or packing it customer orders. So like all of these tasks that require handling of goods are being solved by robots today on one AI brain. So, I would say like. In some degree like this chat, the moment for robotics is happening now, right and it’s going to have a slower take off than the language world because it's the language world is purely digital, where you everyone can just go to your browser and then access chatGPT that's not true for AI robotics like because you need physical infrastructure, you need to put a physical robot there. But if you look at it from a technology readiness and from a A perspective, like we actually have enough of that kernel to start the same revolution as it's just the initial take off. It's going to be a little bit slower than robotics, but then language.
Hessie Jones
OK, so tell me about the fleet you were talking about earlier about, you know, building a robotic fleet, so how pervasive is this today when we talk about an individual actually managing a fleet of robots, what does that actually look like?
Peter Chen
So this is a common concept that we have in our deployments of air robotics into the world is in a. In a world where your facility is primarily staffed by people like you would have like a group of people that are doing the individual handling work and then you would have a team leader that oversees a team of people to help them unblock difficult issues and train them. And it's pretty much the same analogy for that in the robotics world, like where we deploy when we deploy a fleet, the robots into customers operations. Like we would have this think of them as robotic team leads like that lead a team of robots that help them troubleshoot difficult issues and help figure out optimal ways to feed the workload to the robots. And so we really think of this as deploying AI robots as an augmentation to the human workforce, right? Like maybe in the past like you can only pack 305 hundred customer orders in one hour. And then you'll be very tired. I'm doing that like in this new world. Like you will be able to lead if later 10 robots and then pack 5000 customer orders in one hour and that's the type of transformation of work that we are seeing. Not that dissimilar from the past, like people. Use pencil and paper to do calculations and then they move to using calculator to do calculation like. It just gives you more capability to do more with the same hour of your time.
Hessie Jones
What do you have any stats? Or any kind of use cases from clients that use your technology that actually talk about the kinds of savings? From either a time perspective or revenue cost savings that they've seen as a result of robotics being in the application.
Peter Chen
Definitely. And that's true for. All the customers that we have right like because if you think about these warehouse operations like they are the places where the cost structure gets scrutinized a lot, right? Because traditionally, warehouses are cost centered, like they're becoming more of a differentiator in capabilities now, but. Like these are the places that you. Would expect a lot of financial scrutiny on how you operate right and so really all these AI robots that we deploy in the world like they need to deliver savings from a labor savings perspective, from an improvement in reliability perspective like these are the things that. You need a strong positive business case for this type of technology to become viable, and this comes back to why you need strong AI like why you need this foundation model that can learn across all these different places so that you can. To deliver unparalleled performance. The reason for that is if you don't do that like you would have a robot that makes you lose money, and then there's no way that we can scale there.
Hessie
OK so I have one last question for you because you are definitely in an industry where things are changing all the time and there is absolutely, I think it'd be ironic if I would say that people can become complacent in a place like yours to be able to say, OK, this is this is awesome that we're going to we're going to actually deliver something that that's going to be transformational for an industry. But you actually live, I would say, in a culture of chaos, where you need to be able to look for the next. That's something like robotics or Covariant can actually solve. So tell me a little bit about your culture and the type of people that work there and how you, you know, how you persist within an industry where things are always changing.
Peter Chen
Yeah, like the concept that. We think about is like how we can build an organization that can repeatedly innovate, right? And that's what we need to do. Like we are now in an industry where you just take the thing that has been done a million times and make it little bit better. Like what we need to do is we actually need to invent new things and we need to do that conconstantly and it makes it comes down to a very deliberate choice on people that we hire, like we hire people that like to challenge conventional belief like we hire people that like to work on core innovation and we need to be very deliberate about culture, right we need to be deliberate in building a culture that really encourage learning, like really encourage learning new things and we need to build a culture where it's normal to attempt to do the impossible things like because all innovations has risks you can. If you're not willing to take risks, if you're not willing to push the boundary of what is normally considered possible, then you cannot innovate and so team and culture sits at the core of how we can build a company that repeatedly innovates.
Hessie Jones
That's great. I have one more question I'd lied. So, Peter, so. What is your goal for 2023 like I do have any major milestones that you're trying to hit this year?
Peter Chen
Yeah, definitely. So as I mentioned before like we're actually sitting at the place where the chatGPT moment for robotics is happening now, and it's at covariant brain, we are rapidly scaling up and deploying robots into multiple industries into multiple customers. And so we see it as a very exciting year. That the AI has become mature enough that we can start scaling this into the world like so. After 2023 like we think we'll look back and say, oh, wow, like that's become so clear that like, this one general AI can help robots be successful over a wide set of customers and use cases and they all learn from each other and improve and that paved the way for even more rapid scale up of their robotics. Into the world.
Hessie Jones
OK, that's great. Thank you so much. And I appreciate you coming on. I know we missed our interview at collision, but this has been fantastic, and I can't wait to follow your success and see where it goes in the coming years. So thank you.
Peter Chen
Yeah. Thank you. For all the good questions. And then I definitely enjoy being here.
Hessie
Thank you. And for our audience, if you have any ideas for topics that you want us to cover in the future, please contact us at communications at altitudeaccelerator.ca. In the meantime, we'll be back next week and have fun and stay safe.
Creators and Guests
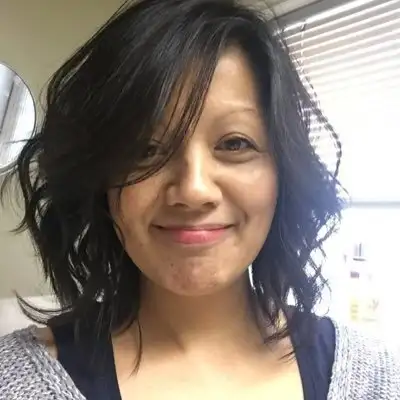
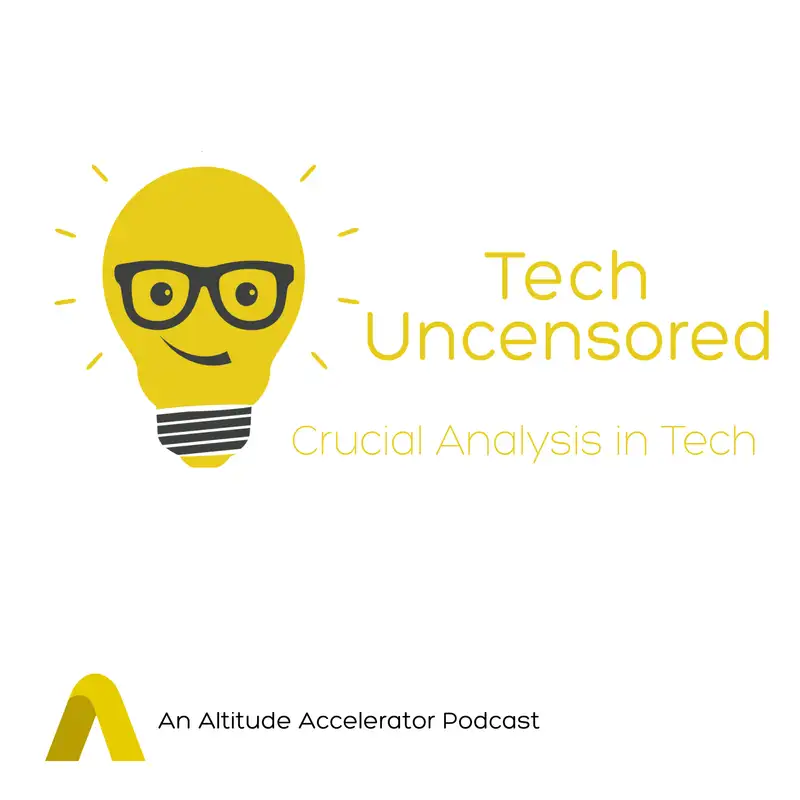