Episode 30 As AI Ubiquity Looms, CAST AI Balances Cloud Efficiency & Environmental Impact
Download MP3Hessie Jones
Hi everyone. I'm Hessie Jones and we're back at Tech uncensored. We're at collision and this is Day 2 and I am happy today to introduce the CEO Signify Raj Ramanand.
Raj Ramanand
Hi, thanks for having me.
Hessie Jones
So today we're actually talking about fraud, in a good way. This is like one of my favorite topics, so signified is a company that you say empowers fearless commerce and Signify uses big data machine learning to provide 100% financial guarantee against fraud and chargebacks and approve orders. So let's talk a little bit about exactly what your solution does.
Raj Ramanand
If you look at the world of retail today, when somebody buys something online and if it turns out to be a fraudulent transaction, retailers have the full liability for that. What that means is that retailers have started putting a lot of protection in place. They add friction across the customer journey to be able to manage the liability for fraud and in return for doing that, they end up turning away or declining millions and millions of dollars of good sales. So ultimately the retailer, in response to that 1% of fraud is turning away somewhere between 10 to 15% of good transactions. What we do at Signified as we come in and say something like, hey, is this transaction good or bad to the retailer and if we say it's good, ultimately the retailer. Ends up being fully covered by us with =the protection in place. So, what we enable for retailers, this massive conversion on the top line while protecting them on the downside.
Hessie Jones
OK, so I'm going to go back a little bit. There was a. Study done by cybersecurity ventures and they said global cyber-crime costs are expected to reach 10.5 trillion U.S. dollars annually by 2025, and this was up almost 3 trillion from 2050. This represents one of the greatest transfers of economic wealth in history and not in a good way. So they even talk about the fact that cyber-crime cost organizations and incredible 1.9 million every single minute. So, I used to work in banking. Between the merchant and the financial institution, typically, who bears the cost, like how does that mechanism work in today's world when there is a transaction?
Raj Ramanand
Just to make it simple, if I steal your credit card and I try to buy something from a retailer online, there are three parties in the mix me, the consumer, the retailer and then the bank. In a card in our present world where somebody buys something online, the retailer takes full liability for that fraudulent transaction, so the banks are protected and the consumers are protected completely on the outside. So that's how the flow works.
Hessie Jones
What are you doing specifically to help? Retailers, I guess optimize the revenue stream and mitigate the downside risks.
Raj Ramanand
Yeah. So the best way to think about this is imagine today retailers are trying to protect about 1% in total fraud that comes in when you think about the amount of transactions, they decline for that, it's somewhere in the range of 10 to 15% of good traffic. If you sort of map that back to a physical store, what that would sound like is for every 10 new customers, you get into the store, you're turning away one customer from coming in. In the physical world, that would be appalling to someone to say. Let's do it and so for in the online world, it seems like it's OK practice. What happens in today's world and the reason for those declines is because most retailers use traditional rules-based systems, you know, so give you an example. Somebody says the IP address came from Vietnam, it's shipping. The Mexico City at 2:00 AM in the morning, human being writes that rule, and that rule sits there and manually says I'm going to decline this transaction or turn away this transaction, but there's a lot of good reasons that somebody could be travelling, or somebody could be shipping it as a gift to someone and that doesn't mean they're a bad person. What we do at signify it is we have data across thousands of retailers where we study patterns of behavior, and we can identify across our 600 million plus wallets if somebody if some pattern of behavior is good or bad. And we can step into the mix for the retailer as that transaction is happening and saying, hey, retailer, this transaction looks perfectly fine, you don't need to decline. To them, and if it turns out that we do make that mistake, as I mentioned earlier, we end up taking full liability. So that's how we sit in the mix and that’s how we do it.
Hessie Jones
Let's take an example of, you know, phishing is becoming more commonplace today. Somebody could go into generative AI and say, hey, write me a text message that sounds really appealing, but legitimate to a user so that they could redeem some reward cards. If that's taking place more often, can your solution actually adapt to those kinds of situations?
Raj Ramanand
This is the AI versus AI debate a little bit, but one of the interesting examples, I mean, we've all grown up with this. I'm sure you've dealt with it where you've dealt with the Nigerian Prince sending you an e-mail and saying I'm trapped somewhere. I need $200.00 and you know, our generation probably didn't react so much to it, but the older generation sometimes, like my mom, would probably click it and say, hey, this is interesting, I’m going to help this person but ultimately what happened is the emails used to be so badly written that most people would recognize it and say this e-mail that came out has grammatical errors, this mustn't be real. But with Gen AI, what's happening Is anybody sitting in a little cafe in Nigeria, let's say can easily go into a ChatGPT model. And say something like, hey, write me an e-mail that's coming from, say, one of the best customer service agents out there saying you need to log into your and complete this transaction and if that goes out as a phishing e-mail to my mother, she's going to believe it and trust it because it looks authentic and she's going to click in type her login and password into that bank account or that merchants website and they've got that information. A large portion of what's happening with AI is that the tools are not just available anymore for the good people that are available for the bad, and they're using that a lot more to be able to commit fraud. Signified systems are built to be able to manage for that, simply because we are not looking at one thing that changed or one piece of text. We're looking at thousands of variables on a transaction in real time and the machine is making the same prediction. It's saying, hey, something is wrong. Raj tends to log in from here. He tends to buy here. He tends to ship here and his patterns of behavior, looking in a certain way across transactions. We're not looking at the individual identity of that person, but we're looking at the behavior of transactions, and therefore we can predict with ease that, hey, this particular transaction is not looking good. From a retailer's perspective, even if we don't get it right every time, if we make that one mistake, we're going to cover them for it.
Hessie Jones
Let's go a little bit deeper into your technology, specifically the AI component. You use real time order scoring as well as identity verification and device fingerprinting.
Raj Ramanand
You know big picture in in AI and machine learning, the feedback loop is a super important part of how a machine. You can learn especially in transactional data sets when you have Gen AI sort of large language models. It's learning across language and context, not necessarily always with the feedback loop. In the traditional transactional models, you need to have that feedback loop. One of the beautiful parts of our business is we commit to paying you back 100%. If we make a mistake and that means, it's in the best interest of every retailer to tell us when we make a mistake because we're going to pay them back in cash. Because we do that, we get that strong feedback loop almost instantly and with that feedback loop we can train models at scale, so you know there's a lot of point solutions in the market like device fingerprint or somebody else is doing an address lookup. We sort of look at transactions holistically across many different variables. Thousands and thousands of variables and train models to be able to learn what is good and bad based on that outcome or that feedback loop we get, and by doing that we can predict where something doesn't look. Right, because the machine has been able to look at so many different signals to be able to make that decision. So going back to your question, those point solutions are valuable inputs, but if knitted together they make the holistic solution to be able to make the prediction.
Hessie Jones
Perfect. I always say that any big institution is always complacent. They're slow to adopt these things. What kind of traction have you had and what advice would you have for retailers and brands who are experiencing this kind of stuff and they want to mitigate it, but they also want to transform in a way that that makes it easier for their customers?
Raj Ramanand
Two big trends have led to the scale at which we are today looking at 600 million plus wallets across the globe is a pretty massive number. When we look at a transaction for the first time, when a retailer sees it for the first time, we've seen it 98-99% of the time. We've seen someone coming in to buy and that's a massive amount data that allows us to make these predictions. But there are two trends that are really driving that scale and growth. The number one trend is we will continue to see a push into digital, that's just going to be the natural way people are going to buy over time. This accelerated with the pandemic. You saw a lot of companies pushing into the online world and trying to figure out how do I be able to sell more frequently, it could be there was the rise of buy online, pick up in store which was going and so there was this whole push into digital that was #1. The second wave is coming through with the way the economy is positioned right now and it's going to be that way for a little bit of time is that as the economy worsens, you start seeing a lot of growth in fraudulent behavior from first party buyers. You know, these are good buyers but have some level of buyer's remorse, more frustrations with working with the company. So as an example, you see people buy something, say tickets to a concert, and they come back after the concert and notice that it was $2000 for six tickets. I'm going to file a charge back and say I never did the transaction. You're seeing people who buy something and then it gets delivered to their house like say, an iPhone or an electronics item or anything else, and then claimed they never received it, even though it was delivered to their house because it was hey, this was 600 bucks. You know if your policies are easy, you're seeing the last one. I'll sort of give you as an example, is with the rise of easy customer policies that have been built over the years. I bought something and I know your return policy is 30 days out. I'm going to wear it for two or three weeks and then come back and return it. It's called wardrobing as a way and you're starting to see all these abusive behaviors grow that merchants can't separate. What's good behavior from bad behavior? It's easy to go back and say I'm not going to let this person buy it because they've returned it twice, but do you really want to do that? Because that person may have just returned it for good reasons, but their lifetime value might be a lot higher. And that's where I think you'll continue to see the massive growth in needing to separate what is good from what is bad and being able to do that on a scale as you continue to build your business and scale your retail business.
Hessie Jones
That seems almost difficult to do, basically saying that a person is good in 90% of the cases. But sometimes you know they just want to save a little bit of money. Is that something that you could actually separate or or identify?
Raj
This is the power of having thousands of retailers within the network. You start to see buying behavior. You start to see when people do certain things. You obviously want to make it easy for the customer to be able to do things with you by taking out the friction from the process. But you also want to be able to identify which ones are not going to be great lifetime value customers for you. And as a retailer that's out there today and growing my business, the best way to scale is to be able to grow your existing customer base and value tremendously. When you get a new user because you want to make their experience beautiful, and that's where we come in and signify to be able to help you identify. Which ones of these are really valuable and how you should be able to allocate the right structure to support them in the right way.
Hessie Jones
Great. Thank you. So one last question, what does 2023 look like for you?
Raj Ramanand
2023 has a couple of great things in the works. One is the changing trend. As I mentioned, account abuse is on the rise for us in the retail space as a whole and what that means is the demand for signified services being really important to them. The 2nd is just with the economy going the way there is, there's a big push on cost and one of the things signify does tremendously well is reduce your cost of ownership. So, if you think about fraud in general and I started. This and sort of I think it's valuable about 10 to 15% of all orders that people turn away today are good. When you think of that in the scheme of things, that's $400 billion on an annual basis that's being turned away in the economy. What our role is in the world today is to be able to continue to put that 400 billion back into the world. 400 billion back into the world simply because that helps the economy continue to grow. But on the other hand, it's also to take out the cost, the cost of hiring, 50 new engineers, 100 new data scientists, 200 new rules writers. These are costs that businesses have to manage if they have to manage it themselves. Not only do we help them grow their top line, but we help reduce some massive costs so you can repurpose people to be able to work on different problems that are core to the business and let fraud not be one of those. And by doing that, we can really pay for a lot of the business elements that they have today that they need to grow by being able to implement a solution like us while also getting that guarantee that if something turns out to be bad, we'll cover it. 2023 is all about that cost reduction for retailers so that they can continue to scale. Being ready for this account abuse wave and then continuing to help these guys grow their top line.
Raj Ramanand
Perfect. Thank you. Well, good luck this year and thank you for joining me today.
Hessie Jones
Wonderful. Thanks for.
Raj Ramanand
Having me, thank you.
Creators and Guests
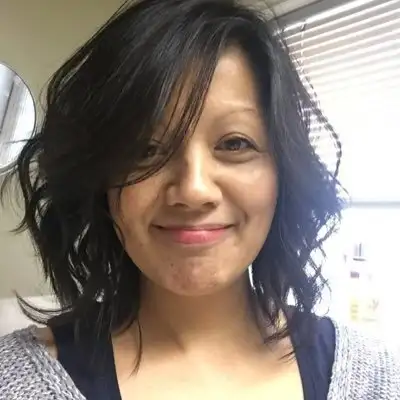
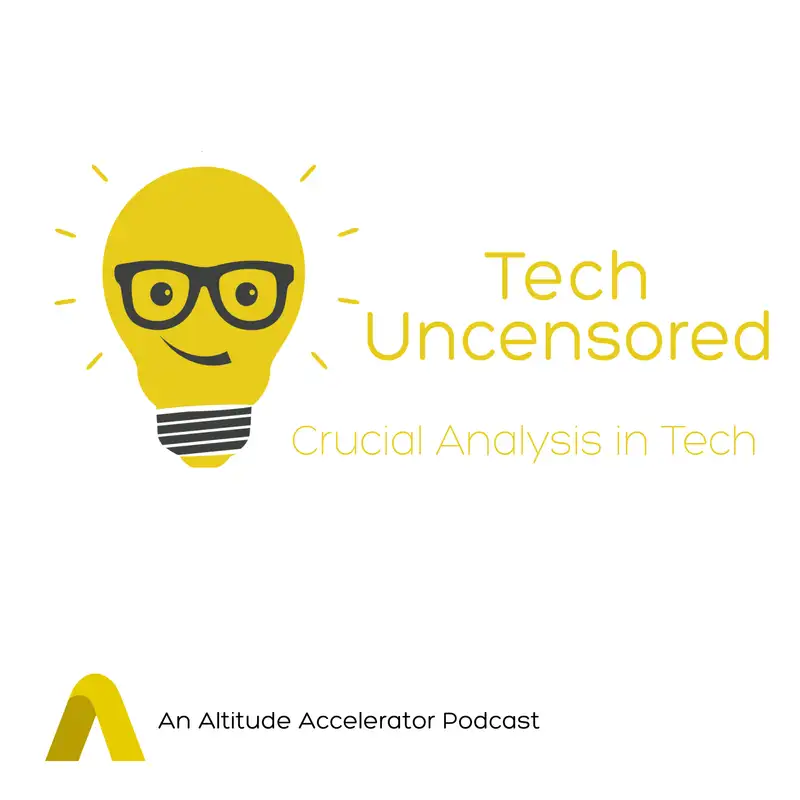